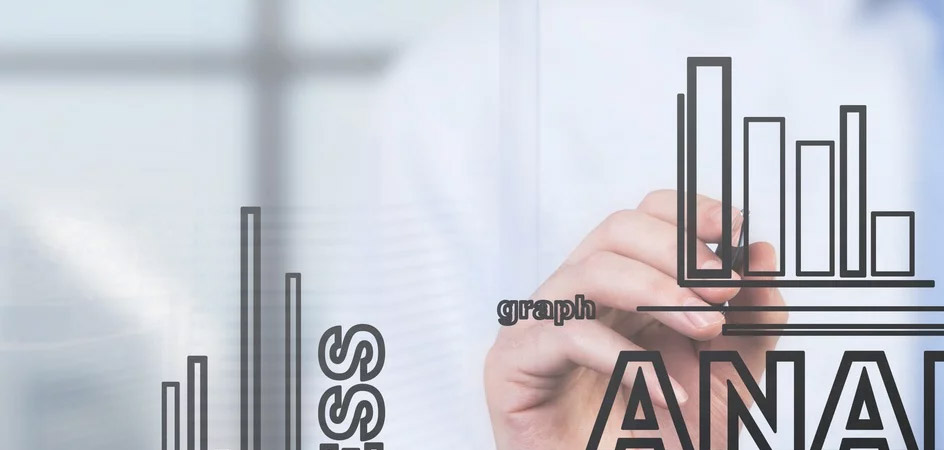
Differences Between Centralized And Decentralized Analytics

Posted by Walid Abou-Halloun Date: Dec 28, 2017 3:33:04 AM
To centralize or not to centralize? That is the question when debating centralized vs decentralized analytics.
But the trade secret of analytics structures like these is that they’re only just beginning their impact as a competitive resource – meaning your business has time to learn the ropes and get ahead.
How do you choose between centralized and decentralized analytic structure? Or, rather, how do you decide what level of centralization is right for your business? Keep reading to find out the basics, benefits, and trade-offs of both sides.
What is Centralized Analytics?
As the name suggests, everyone sticks together.
Basics of the Model
In a fully centralized analytics structure, a group of analysts functions as a core unit to serve the whole company. In a pure centralized analytics case like this, the analytics team is their own entity that may report to IT, strategy or, in a more popular option, make up their own C-suite under a Chief Analytics Officer.
This model is most successful – and most popular – among large companies that are a single-business model with a strong need for analytics.
Variants of the Model
As easy as it is to think of centralized vs decentralized analytics as two opposing poles, that isn’t exactly the case. In fact, there are a few grey areas between the two.
Here are the two closest to the strictly centralized model.
The Consulting Model
Like the centralized model, the consulting model involves a core group of analysts.
Unlike the purely centralized model, however, analysts in the consulting model are tasked with analytics projects throughout the whole company. They make up for their costs by charging for their time on those analytics projects.
Think of the consulting model kind of like your company IT guy. He’s not there solely to fix computers in your part of the office – instead, he fixes things as he’s needed to fix them, wherever he’s needed to fix them.
Same idea here.
That said, the major drawback in this model is the strong temptation for the company to follow the money instead of allocating analysts where they’re most needed.
The Centre of Expertise Model
As analytics models go, the centre of expertise model sits somewhere in the middle of the centralized vs decentralized analytics scale.
Think back to our example of the consulting model analyst as your company IT guy. Remember how we said that IT guy is a floater – drifting between departments instead of staying within your department?
In the centre of expertise model, the analysts aren’t floaters anymore. Instead, they’re based in business functions and units, and their actions are coordinated by a small centralized group.
The benefits are as obvious as the downsides. While your analysts have a better chance to be where they’re needed, the centralized unit may have trouble managing the actions of decentralized analysts, which can make it more difficult to control for effectiveness.
What is Decentralized Analytics?
If you can follow the trend of the centralized vs decentralized analytics scale, you can probably already guess the defining characteristics of the decentralized model.
In any case, the name tends to give it away.
Basics of the Model
In a purely decentralized analytics model, the analysts work directly for individual departments rather than any centralized unit coordinating their actions.
Knowing what we know about centralized analytics, the benefits of a decentralized system are obvious – departments aren’t competing for the analysts’ attention, and a central department isn’t struggling to coordinate them.
Variants of the Model
Outside of the purely decentralized, or dispersed, analytics model, the only other model on the centralized vs decentralized scale that comes close to a decentralized system is called the functional model.
The Functional Model
The simplest way of explaining the functional model is to say that analysts are put right in the middle of the action.
In the functional model, analysts aren’t shared throughout the company or specialized to one department under a central analytics unit. Instead, they’re all placed where the most analytical manpower is required.
For example, if the dominating analytical area at a company is financial, then all of the analysts will stay within the financial department.
You can figure out the benefits of this system easily – analysts are placed directly where the company deems them most useful. The downside, of course, is that expanding analytics outside that single department is an uphill battle.
Centralized vs Decentralized Analytics
Knowing what we know about the centralized vs decentralized analytics, it becomes easier to point to key differences between them.
But first, a bit of groundwork: as we’ve seen, centralized and decentralized analytics models aren’t a dichotomy. As such, the question for your company isn’t really which model to choose so much as which version of the model to choose, depending on your business model and company needs.
How are They Different?
The first question to ask yourself, which considering centralized vs decentralized analytics, is what you’re looking for in an analytics team.
You should also consider what kind of mobility you’ll need an analytics team to have based on what your business needs are.
If, as we said, your business is based on a single core focus, then a centralized analytics team could suit you. If your company covers several separate areas of business, you could be more suited to decentralized analysts.
Centralized Analytics Benefits
The benefits of a centralized analytics system are all based on the fact that the analysts remain more or less in their own group, or monitored by a central unit responsible for ensuring their performance quality.
The biggest benefit of centralized analytics, therefore, is an approach focused on standards and practices. If your goal is to create an analytics structure in which organizational alignment is critical, then you need a standards and practices approach.
How do you do this? You start with everyone having the same tools, and by making it easy for everyone to share the same strong knowledge base. In this case, you’re better off with a centralized analytics team.
Another benefit is flexibility. Think of it this way – if you have to personally oversee your analysts every time they move between departments when workloads ebb, you’re burning a lot of time.
If on the other hand, the analysts are free to move where they’re most needed, you’re saving everyone a lot of time and hassle.
Centralized Analytics Tradeoffs
Unfortunately, this same flexibility is the downside to a centralized analytics model. That is because your analytics team is drifting to whoever needs them most on any given team, it’s difficult to establish a central, focused analytics objective.
It’s also common that the needs of one department will usurp the needs of another, which is why we see the rising popularity of decentralized analytics teams out of the frustration of departments who get left behind.
This frustration is also where it becomes easy to polarize centralized vs decentralized analytics models. One department has deeper pockets than another. Said department poaches analysts, leaving others behind.
The departments who get left behind hire their own people out of frustration. And out of the blue, you have a decentralized model where you didn’t intend to have one.
Decentralized Analytics Benefits
As you might guess, the benefits of a decentralized analytics team are a response to the issues with a centralized one.
The biggest benefit of a decentralized system is that everyone gets what they need from analysts without having to poach them from other departments or worry about their needs getting the short straw. In this sense, everyone’s happy.
Another plus is that because analysts are staying in one place, they become talented at doing the specific kind of analytics their department needs from them. They also don’t have to worry about their workload covering a thousand different tasks, since they stay loyal to their home department.
Decentralized Analytics Tradeoffs
On the other hand, because there’s no central analytics team for the whole company to draw on, the whole analytics effort can wind up being unfocused. After all, everyone already has their own job to worry about – they’re not going to worry about creating a focused analytics team out of decentralized players.
This is why decentralized analytics teams are much more commonly seen in small companies who don’t have a huge number of analysts. It simply doesn’t work to have a decentralized analytics team when you want your analytics team accomplishing enterprise goals for you.
With this in mind, you should consider how big your company is and how many analysts you actually have when choosing a centralized vs decentralized analytics model.
Better Business, Better Workplace Consultants
So you know all about centralized and decentralized analytics systems – where do you go from here? If you are looking to expand or establish your analytics ecosystem with some Big Data Resources and are looking to find candidates with the precise skill set to drive value throughout the organisation speak to one of our specialised consultants today.
At Divy, our experience is in identifying our client’s existing skills gap, finding the right candidate and successfully placing them within the organisation.
For us, it’s all about the mind-set. Courting our partners doesn’t stop once ink goes to paper – we make the process smooth and maintain high standards of quality all throughout our partnership, from the candidate that is selected, to how we monitor performance and the way we provide support.